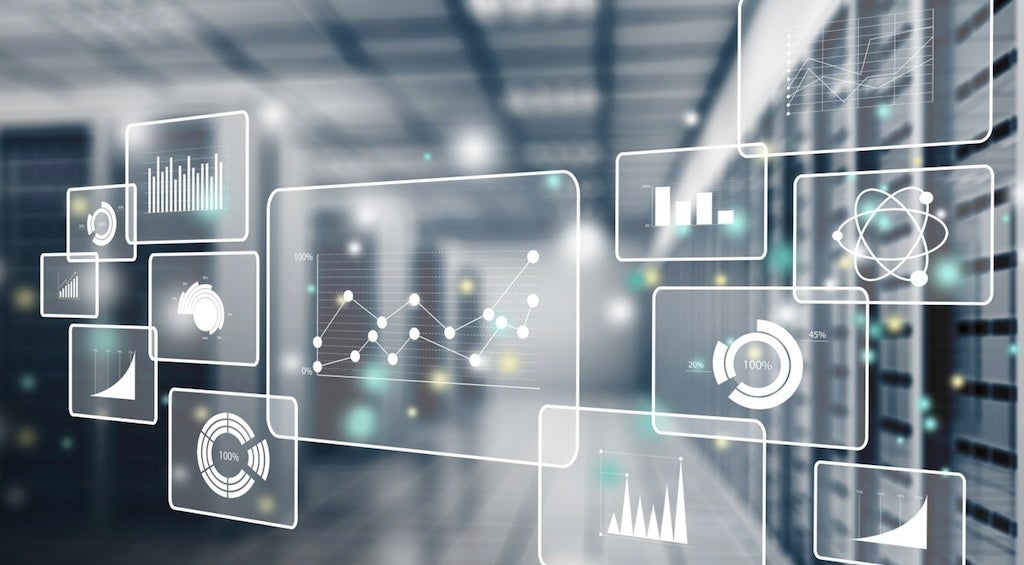
In today’s data-driven world, organizations increasingly rely on data to make informed decisions. However, the utility of this data is directly proportional to its quality. Data quality is not just a buzzword; it’s a critical aspect that can make or break business strategies, and research outcomes, and even influence policy changes. Let’s delve into the intricacies of data quality and discuss why it’s essential to ensure data accuracy and relevance.
What is Data Quality?
Data quality refers to the condition or state of data in terms of its fitness to serve its intended purpose in each context. Essentially, it is about how accurate, complete, reliable, relevant, and timely the data is for the task at hand. Here is a breakdown of some key dimensions that are often considered when talking about data quality:
Key Dimensions of Data Quality
- Accuracy: The data accurately represents the real-world entities or events it is supposed to model. Incorrect or outdated information can lead to faulty analyses and decisions, thereby negating the purpose of using data in the first place.
- Consistency: Data is uniform across different datasets or time periods, allowing for seamless integration and comparison.
- Completeness: All the necessary data fields are filled in, leaving no gaps that might impair analysis or decision-making.
- Reliability: The data can be trusted as a basis for analysis and decision-making, often achieved through data governance measures and proper sourcing.
- Relevance: The data is applicable to the problem or task at hand. Irrelevant data can skew meaningful insights and may even lead to incorrect conclusions.
- Timeliness: The data is up-to-date and available when needed. Outdated data can be misleading and result in sub-optimal decisions.
- Accessibility: Data should be easily accessible by those who need to use it but secure enough to prevent unauthorized use.
- Uniqueness: Elimination of duplicates ensures that each data entry is unique and non-redundant, thus improving the quality of analyses.
- Integrity: This refers to the structural soundness of the data. Integrity ensures that relationships between different data fields are maintained without corruption.
Understanding and improving data quality is essential for any organization that relies on data for decision-making, analytics, and operational efficiency. Poor data quality can lead to inaccurate analyses, inefficient processes, and, ultimately, the wrong conclusions, which can have a material impact on business or research outcomes.
The Domino Effect of Poor Data Quality
Poor data quality often sets off a domino effect of adverse events:
- Decision-making: Poor quality data can lead to misguided decisions that might cost businesses both time and resources.
- Compliance: Inaccuracy in data can result in non-compliance with legal or regulatory standards, inviting penalties.
- Operational Efficiency: Inconsistent and irrelevant data can disrupt operational workflows, leading to inefficiencies.
- Trust: Faulty data will erode constituent trust, impacting long-term relationships and possibly brand value.
How to Ensure Data Quality
Data Governance
Implementing a robust data governance framework can set the ground rules for data management and quality assurance. This framework will specify who is responsible for what data and establish guidelines for data storage, access, and quality checks.
Regular Audits
Periodically reviewing the data for accuracy and relevance will help in identifying and rectifying errors. These audits can be automated or manual, depending on the complexity and the volume of the data.
Data Quality Tools
Utilizing data quality tools can automate the process of cleaning and validating data. These tools can identify missing values, duplicates, or inconsistencies, making the dataset more reliable. Since my blog is not a platform for advertising specific products, I encourage readers to explore options in this arena, but there are several commercial and open-source tools available. Each tool has its own pros and cons, and the best fit for you will depend on your specific needs, the types of data you are working with, and the scale of your operations. Always consider factors such as scalability, ease of use, integration capabilities, and cost when selecting a data tool.
Conclusion
The importance of data quality cannot be overstated. Accurate and relevant data is the backbone of informed decision-making, compliance, operational efficiency, and customer satisfaction. Investing in data quality is not just a technical requirement but a critical business strategy that pays off in both the short and long term.
So, whether you are part of a large unit or a small research team, remember that the quality of your data will ultimately define the quality of your outcomes.
To qualifying your decisions through data!
Brian M. Morgan
Chief Data Officer, Marshall University